Developing risk prediction models using nested case-control data: methods and applications

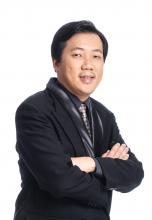
In the last 10-15 years, there has been an explosion of prediction models developed to predict risk of various diseases. The main objective of a risk prediction model is to predict the absolute risk of a disease. For this reason, cohort study design has been used in almost all studies that build or compare risk prediction models because cheaper alternatives such as case-control study cannot be used to validly estimate absolute risk.
In this talk, I will examine the feasibility of estimating absolute risk using nested case-control data. Two approaches will be compared using both a simulated and real dataset from a nested case-control study of coronary heart disease conducted within the Singaporean Chinese Health Study (SCHS) cohort. Finally, I will demonstrate how the methods can be used to investigate the usefulness of two biomarkers, highly-sensitive C-reactive protein (CRP) and serum creatinine to improve prediction of coronary heart disease (CHD) risk, on top of the traditional risk factors used by the Adult Treatment Panel III (ATP-III) risk calculator.