If calibration, discrimination, lift gain, precision recall, F1, Youden, Brier, AUC, and 27 other accuracy metrics can’t tell you if a prediction model (or diagnostic test, or marker) is of clinical value, what should you use instead?
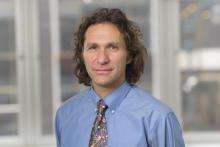
A typical paper on a prediction model (or diagnostic test or marker) presents some accuracy metrics - say, an AUC of 0.75 and a calibration plot that doesn’t look too bad – and then recommends that the model (or test or marker) can be used in clinical practice. But how high an AUC (or Youden, or Brier or F1 score) is high enough? What level of miscalibration would be too much? The problem is redoubled when comparing two different models (or tests or markers). What if one prediction model has better discrimination but the other has better calibration? What if one diagnostic test has better sensitivity but worse specificity? Note that it doesn’t help to state a general preference, such as “if we think sensitivity is more important, we should take the test with the higher sensitivity” because this does not allow to evaluate trade-offs (e.g. test A with sensitivity of 80% and specificity of 70% vs. test B with sensitivity of 81% and specificity of 30%). The talk will start by showing a series of everyday examples of prognostic models, demonstrating that it is difficult to tell which is the better model, or whether to use a model at all, on the basis of routinely reported accuracy metrics such as AUC, Brier or calibration. We then give the background to decision curve analysis, a net benefit approach first introduced about 15 years ago, and show how this methodology gives clear answers about whether to use a model (or test or marker) and which is best. Decision curve analysis has been recommended in editorials in many major journals, including JAMA, JCO and the Annals of Internal Medicine, and is very widely used in the medical literature, with approaching 1500 empirical uses a year.
Andrew Vickers is a biostatistician and attending research methodologist at Memorial Sloan Kettering Cancer Center and professor of public health at Weill Cornell Medical College. Dr. Vickers’ methodological research centres primarily on novel methods for assessing the clinical value of predictive tools, having developed the statistical method known as "decision curve analysis". He has a strong interest in teaching statistics and is course leader for the MSK biostatistics course and author of the introductory textbook “What is a p-value anyway?"
Thursday 27th October
9-10am AEDT
This event will be streamed via Zoom.
Please click here to join
Or, go to monash.zoom.us/join and enter meeting ID: 88620776350 and passcode: 348708