Causal Inference with Continuous (Multiple-Time Point) Exposures
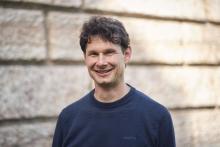
There are limited options to estimate treatment effects of variables which are continuous and measured at multiple time points (particularly if one is interested in the actual dose-response curve). However, these situations may be of relevance: in pharmacology, one may be interested in how outcomes of people living with -and treated for- HIV, such as viral failure, would vary for time-varying interventions such as different drug concentration trajectories.
A challenge for doing causal inference with continuous interventions is that the positivity assumption is typically violated. To address positivity violations, we develop projection functions, which reweigh and redefine the estimand of interest based on functions of the conditional support for the respective interventions. With these functions, we obtain the desired dose-response curve in areas of enough support, and otherwise a meaningful estimand that does not require the positivity assumption. We develop g-computation type plug-in estimators for this case. Those are contrasted with g-computation estimators which are applied to continuous interventions without specifically addressing positivity violations, which we propose to be presented with diagnostics.
All ideas are illustrated with longitudinal data from HIV positive children treated with an efavirenz-based regimen as part of the CHAPAS-3 trial, which enrolled children <13 years in Zambia/Uganda. Simulations show in which situations a standard g-computation approach is appropriate, and in which it leads to bias and how the proposed weighted estimation approach then recovers the alternative estimand of interest.
Additionally, possible extensions of the proposed approach, as well as relevant software packages are introduced briefly.
Michael Schomaker is a Professor for Biostatistics working under the German Research Foundations Heisenberg Program at the Department of Statistics, Ludwig-Maximilans-Universität München, Germany. He is also affiliated with the University of Cape Town, South Africa and UMIT University, Austria. His interests cover a wide range of topics including causal inference for imperfect longitudinal observational databases, missing data, causal fair machine learning, and infectious disease epidemiology. Besides doing research, he teaches in the Master of Statistics & Data Science degree programme at LMU Munich.
This presentation will be held over Zoom and will be recorded.
Please click this URL to start or join:
https://monash.zoom.us/j/88957888875?pwd=L3BwZlJnUEkzQ2RZYU1BZjBmR0Y0Zz09
Or, go to https://monash.zoom.us/join and enter
Meeting ID: 889 5788 8875
Passcode: 820 195