Summer School 2025: Design and Analysis of Sequential, Multiple Assignment, Randomized Trials (SMARTs)
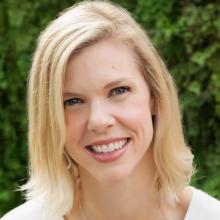
Most diseases and disorders require ongoing, tailored treatment that adapts to an individual’s needs and responses. Yet, evidence for effective treatment is often produced at one point in time. That is, the gold standard of evidence, a randomized, controlled trial (RCT), most often investigates if an intervention is effective in a sample of individuals at one point in their disease or disorder progression. Although the classic RCT is valuable, we may be missing treatment synergies or antagonisms by not investigating more of the intervention process in the same individuals. These effective treatment guidelines that specify whether, how, when or for whom to alter treatment at critical decision points across the scope of care are called dynamic treatment regimens (DTRs). One way to rigorously develop DTRs is with sequential, multiple assignment, randomized trials (SMARTs).
A SMART is a type of multi-stage randomized trial design such that some subset of participants are randomized at least twice in sequence. Subsequent randomization and/or treatment assignment is based on response to previous treatment. Within a SMART design, several DTRs are embedded. This workshop will introduce DTRs and describe how SMART designs can be used to develop, estimate and compare high-quality DTRs. SMART designs are contrasted to other similar designs. SMART design principles are discussed along with the typical primary, secondary and exploratory aims, especially considering the needs of grant proposals or trial protocols. The workshop focuses on a high-level overview of analytic methods of individual and cluster randomized SMART designs including analysis for primary, secondary and exploratory aims, along with power and sample size calculations. The designs and methods discussed are illustrated through real case studies, exercises, and illustrated by running provided code in R hands-on coding in R.
Prerequisites and intended audience:
- Some experience in the design of clinical trials
- Good working knowledge of basic principles of statistical inference (e.g., standard errors, hypothesis tests), linear and logistic regression
- Basic knowledge of R would be useful, but not essential. R scripts wlll be provided.
Registration:
Discounted tickets are available for full time students. Please use your student email when registering.
Registration type | Price | |
Standard | $980 | |
Student | $700 |
Registration is now open. MCRI staff and students - to arrange payment via cost centre transfer please email
For all other registrations please visit: https://shop.monash.edu/vicbiostat-summer-school-2025.html
Location:
This course will be delivered in person and online via Zoom.
Monash University SPHPM
553 St Kilda Road, Melbourne 3004
Presenter:
Professor Kelley Kidwell, School of Public Health, University of Michigan, USA
Dr Kelley Kidwell is Professor of Biostatistics at the University of Michigan, USA. Her interests are in the design and analysis of clinical trials, with her methodological work centering on better matching the way in which we practice medicine and public health (critical decisions over time tailored to individuals) to the way in which we experimentally study it in randomised trials.
Dr. Kidwell's methodological work has primarily focused on the design and analysis of sequential, multiple assignment, randomized trials (SMARTs) in standard or large size trials for treating common diseases and disorders, and in small samples or for treating rare diseases. Collaboratively, Dr. Kidwell aims to improve public health science by bridging the gap between researchers, the biostatistical methods needed and applied to studies, and the communication of results.
She is involved in the design and analysis of many trials in settings such as mental health, chronic pain, substance use, and oncology. Her methods and collaborative work influences clinical trial statistical theory and practice with the aim of improving people's lives through new designs and efficient treatment effect estimates.